Matthew Mapa
Aspiring Data Scientist/AI Engineer
Email: matthewmapa@ufl.edu
Hi, I'm Matthew (call me Mapa), a computer science and statistics student at the University of Florida. I've always been drawn to the potential of data to drive positive change. Since coming to the University of Florida, I've worked on several projects aligned with this interest in various domains, and I've gained experience at both startups and larger enterprises. My professional and research interests lie in the applications of data science and AI towards critical environmental and social issues.
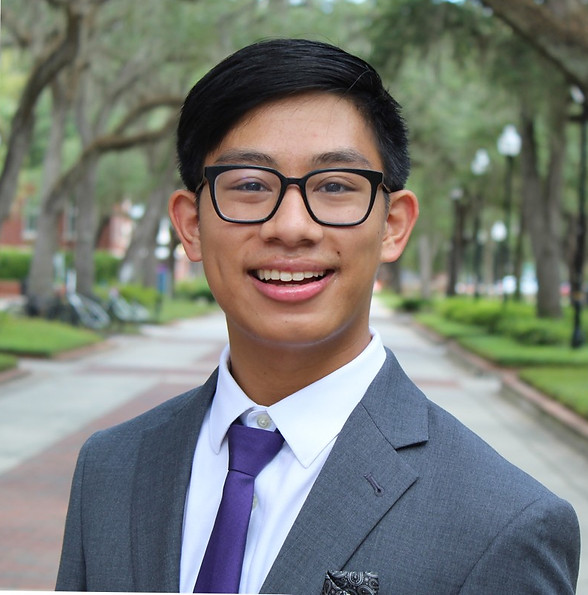
Evacuation Route Choice during
the 2021 Marshall Fire
Principal Investigator: Dr. Xilei Zhao, Department of Civil and Coastal Engineering at the University of Florida
Status: Submitted to the Transportation Research Board for presentation, journal version in progress
August 2023 - Present

Research Focus
Due to climate change and urban development, wildfires are becoming increasingly severe, necessitating that communities create effective and proactive emergency plans. To enhance these plans, understanding household evacuation behavior is critically important to address the challenges of emergency evacuation and accurately and realistically evaluate planning strategies. This project seeks to improve our knowledge of household evacuation behavior by understanding evacuation route choice (i.e., the paths evacuees take when evacuating during emergencies) using large-scale GPS data collected from mobile devices. Our results will help improve emergency plans and make future simulations of evacuation behavior more realistic.
Project Responsibilities
As part of this project, I conducted a literature review to understand existing research on evacuation route choice, simulations for traffic during wildfires and other emergencies, and methods for preprocessing and cleaning GPS data. I developed a methodological framework for processing the large-scale GPS dataset, estimating route choice from GPS data, and comparing the results of our project with the existing assumptions used by evacuation simulations. I generated the results of the study, developed several figures to visualize these results, and evaluated how our results compare to the existing literature. Through this project, I have gained a significant understanding of traffic modeling and working with GIS data while applying my prior knowledge in Python in a new domain. I have also been able to serve as the primary author of the study.

Synthetic example of an evacuation route estimated using our methodological framework compared to the shortest paths by travel time and travel distance
Coursework
Major: Computer Science
Minor: Statistics
Special Distinctions: AI Scholars Program - 2024 Cohort,
Benacquisto Scholar, Presidential Platinum Scholar
Computer Science/Artificial Intelligence
Data Structures and Algorithms
Linear Algebra
Software Engineering
Introduction to Data Science
Database Systems
Machine Learning
Operating Systems
Natural Language Processing​
Statistics
Regression Analysis
Statistical Modeling
Experiment Design
Data Science Intern - Climate and Sustainability Data and Analytics
Truist
Atlanta, GA
-
Reported GHG emissions across over 5000 Truist properties by developing data quality process with team and creating a dashboard using Tableau and SAS, supporting a 35% reduction in Scope 1 and 2 emissions by 2030
June - August 2024
Data Science/Machine Learning Intern
Satlantis, US
Gainesville, FL
-
Researched and developed a model for methane detection using GEISAT multispectral bands, achieving a minimum
detection threshold of less than 100 kg/h and helping secure a multi-million dollar contract -
Augmented model performance to detect over 75% of high-flow rate methane plumes and advancing methane
detection research by engineering a pipeline for simulating methane plumes in satellite images -
Implemented a deep learning model to detect over 90% of pixels containing clouds to expedite ground analysis by
developing an augmented U-Net convolutional neural network in PyTorch -
Enhanced model performance to enable training on over 65,000 images by developing PyTorch Lightning data
modules and customizable data augmentation pipelines for the 95-Cloud and Sentinel-2 datasets
January - May 2023,
August 2023 - May 2024
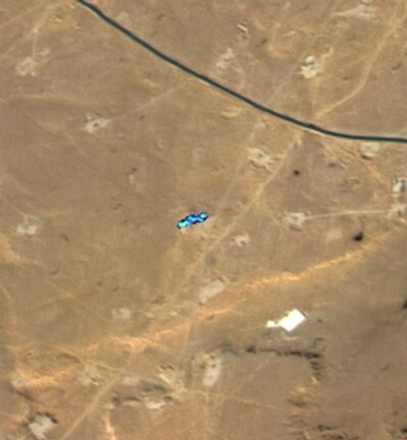
High-resolution image of an 818 kg/h methane emission detection in Africa captured by SATLANTIS’ GEISAT satellite in February 2024
Data Analytics Intern
RVO Health
Charlotte, NC
-
Analyzed driver performance in skincare content to increase engagement clicks by 76% by optimizing over 134
million user journeys using a machine learning model -
Investigated audience characteristics in weight loss content to provide insights into over 51 million user journeys to
inform upcoming app launch by using Python and data science techniques
June - August 2023